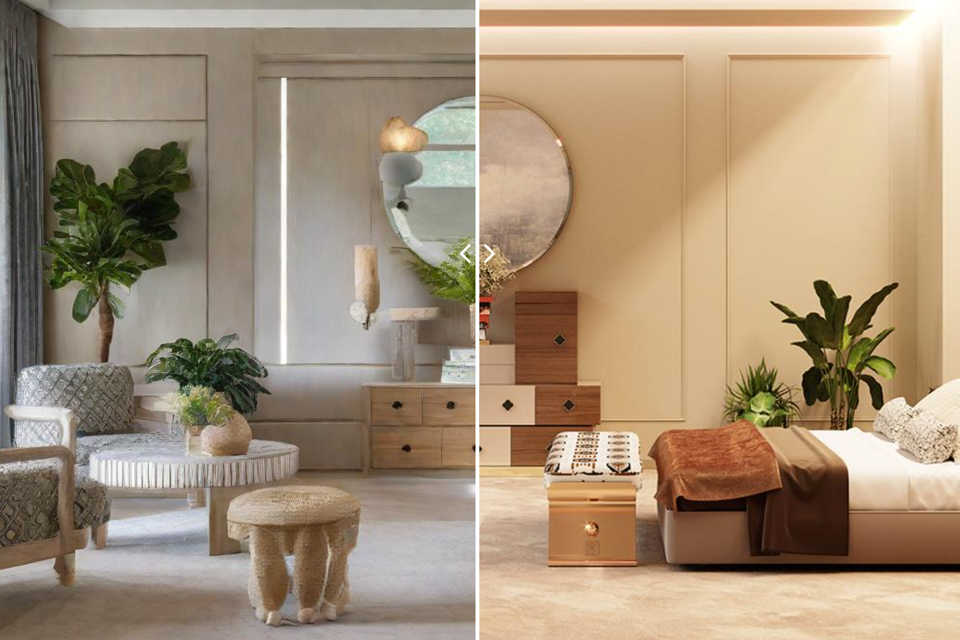
In today's digital age, the marketing landscape is undergoing a dramatic transformation. Data Science, Machine Learning, and Artificial Intelligence are at the forefront of this change, revolutionizing how brands connect with their customers. Let's explore this evolution and the key techniques shaping the future of marketing.
The Journey from Traditional to AI-Driven Marketing
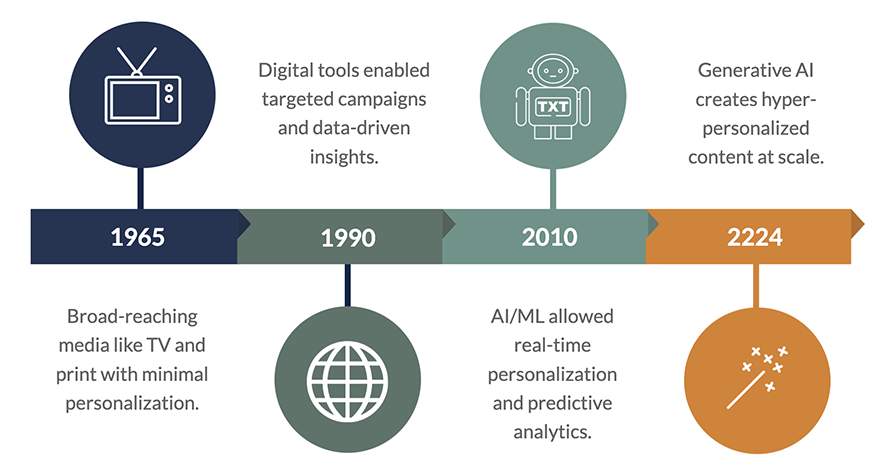
The Pre-AI Era
Remember the days of print ads, TV commercials, and radio jingles? That was marketing in the pre-AI era. Brands cast wide nets, hoping to catch potential customers with little personalization. Marketing strategies relied heavily on broad demographic insights and mass media channels:
- Print advertisements in newspapers and magazines
- Television commercials during prime time
- Radio spots throughout the day
- Direct mail campaigns
- Coupon distribution
While these methods reached large audiences, they lacked the precision and personalization that today's consumers expect.
Statistic: In 1965, a typical consumer was exposed to about 500 ads per day. By contrast, today's consumers are estimated to encounter between 4,000 to 10,000 ads daily. (Source: Spyce Media)
The Digital Revolution
The 1990s ushered in digital marketing, marking a significant shift in how brands interacted with their audiences. Suddenly, marketers could target audiences more precisely and measure campaign impact accurately. This era introduced:
- Email marketing campaigns
- Social media platforms for brand engagement
- Search engine marketing and optimization (SEM and SEO)
- Website analytics and user behavior tracking
- Online advertising with clickthrough tracking
These digital tools allowed marketers to collect vast amounts of data on customer preferences, behaviors, and feedback. However, by the early 2000s, the sheer volume and complexity of this data began to overwhelm traditional analysis methods.
Statistic: Digital advertising spending worldwide reached $667.6 billion in 2024 (Source: Spendesk)
Enter AI and Machine Learning
By the early 2010s, AI and ML began transforming marketing. These technologies enabled unprecedented levels of personalization and efficiency, allowing marketers to predict customer needs and behaviors with remarkable accuracy. Key advancements include:
- Big data analytics for customer insights
- Predictive modeling for customer behavior
- Real-time personalization of content and offers
- Automated customer segmentation
- Chatbots and virtual assistants for customer service
AI and ML helped marketers move beyond basic demographic targeting to create highly personalized customer experiences, predicting needs and behaviors more accurately than ever before.
Statistic: According to a study by McKinsey, AI-driven campaigns have resulted in a 28% increase in revenue and a 23% decrease in marketing costs (Source: WifiTalents)
Core Data Science Techniques in Modern Marketing
1. Predictive Analytics
Predictive analytics helps forecast customer behaviors, from email open rates to purchase likelihood. It's a game-changer for resource allocation and campaign design. Some applications include:
- Email opening likelihood: Predicting when customers are most likely to engage with email campaigns.
- Purchase/conversion likelihood: Identifying which customers are most likely to make a purchase.
- Customer churn prediction: Detecting customers at risk of leaving and implementing retention strategies.
- Website login frequency modeling: Understanding user engagement patterns to inform feature development.
- Contact frequency modeling: Determining the optimal frequency of customer outreach.
Case Study: At the core of Netflix's success is its highly effective recommendation system that analyzes vast amounts of user data to predict and suggest content that individual users are likely to enjoy. (Source: edujournal)
A/B Testing and Experimentation
A/B testing allows marketers to compare different versions of marketing assets, using data to determine which performs better. This scientific approach to optimization involves:
- Creating two versions of a marketing asset (e.g., email subject lines, landing pages, ad copy)
- Randomly dividing the audience and exposing each group to one version
- Measuring key performance metrics (e.g., click-through rates, conversion rates)
- Analyzing results to determine which version performs better
- Implementing the winning version and iterating for continuous improvement
Statistics: Improved User Experience (UX) design resulting from A/B testing can potentially boost conversion rates by **400%** (Source: (Enterprise Apps Today)
3. Customer Segmentation
Data science techniques like cluster analysis help divide broad customer bases into smaller, more targeted groups. This enables personalized marketing efforts tailored to specific audience segments. Segmentation can be based on various factors:
- Demographic data (age, gender, location)
- Psychographic information (interests, values, lifestyle)
- Behavioral data (purchase history, website interactions)
- Customer value (frequency of purchases, average order value)
By understanding these segments, marketers can create more relevant and engaging content, offers, and experiences for each group.
Case Study: McDonald's added items like Fruit & Maple Oatmeal and Southwest Grilled Chicken Salad to cater to the growing segment of health-conscious consumers (Source: BRM)
4. Customer Lifetime Value (CLV) Modeling
CLV modeling helps marketers understand the long-term value of customer relationships, informing decisions on customer acquisition and retention strategies. This involves:
- Analyzing historical purchase data
- Predicting future purchase behavior
- Estimating the total revenue a customer is likely to generate over their relationship with the brand
- Identifying high-value customers for targeted retention efforts
- Optimizing customer acquisition costs based on expected long-term value
Statistic: 20% of customers account for 80% of future revenue (Source: Datategy)
The Future: Generative AI and Beyond
Generative AI is set to revolutionize content creation and customer engagement further. This technology can:
- Create personalized marketing copy at scale
- Generate custom images and videos for ads and social media
- Produce tailored product descriptions based on customer preferences
- Develop chatbots that engage in more human-like conversations
- Create dynamic website content that adapts to individual users in real-time
Imagine dynamically generated marketing materials tailored to individual customer interests – that's the future of personalized marketing.
Case Study: Coca-Cola is using GPT-4 to generate a diverse range of high-quality content. (Source: Hackernoon
Embracing the AI-Driven Marketing Era
As we move forward, integrating AI and data science into marketing strategies is no longer optional – it's essential for brands aiming to stay competitive. This shift represents not just a technological advancement, but a fundamental change in how brands connect with their audiences, offering more personalized, engaging, and meaningful interactions.
To stay ahead in this AI-driven marketing landscape, companies should:
- Invest in data infrastructure to collect and analyze customer data effectively
- Develop in-house data science capabilities or partner with specialized agencies
- Continuously experiment with new AI and ML technologies
- Prioritize customer privacy and data security
- Foster a data-driven culture across marketing teams
The future of marketing is data-driven, AI-powered, and incredibly exciting. By embracing these technologies, brands can create more impactful campaigns, build stronger customer relationships, and drive sustainable growth in an increasingly competitive digital landscape.